
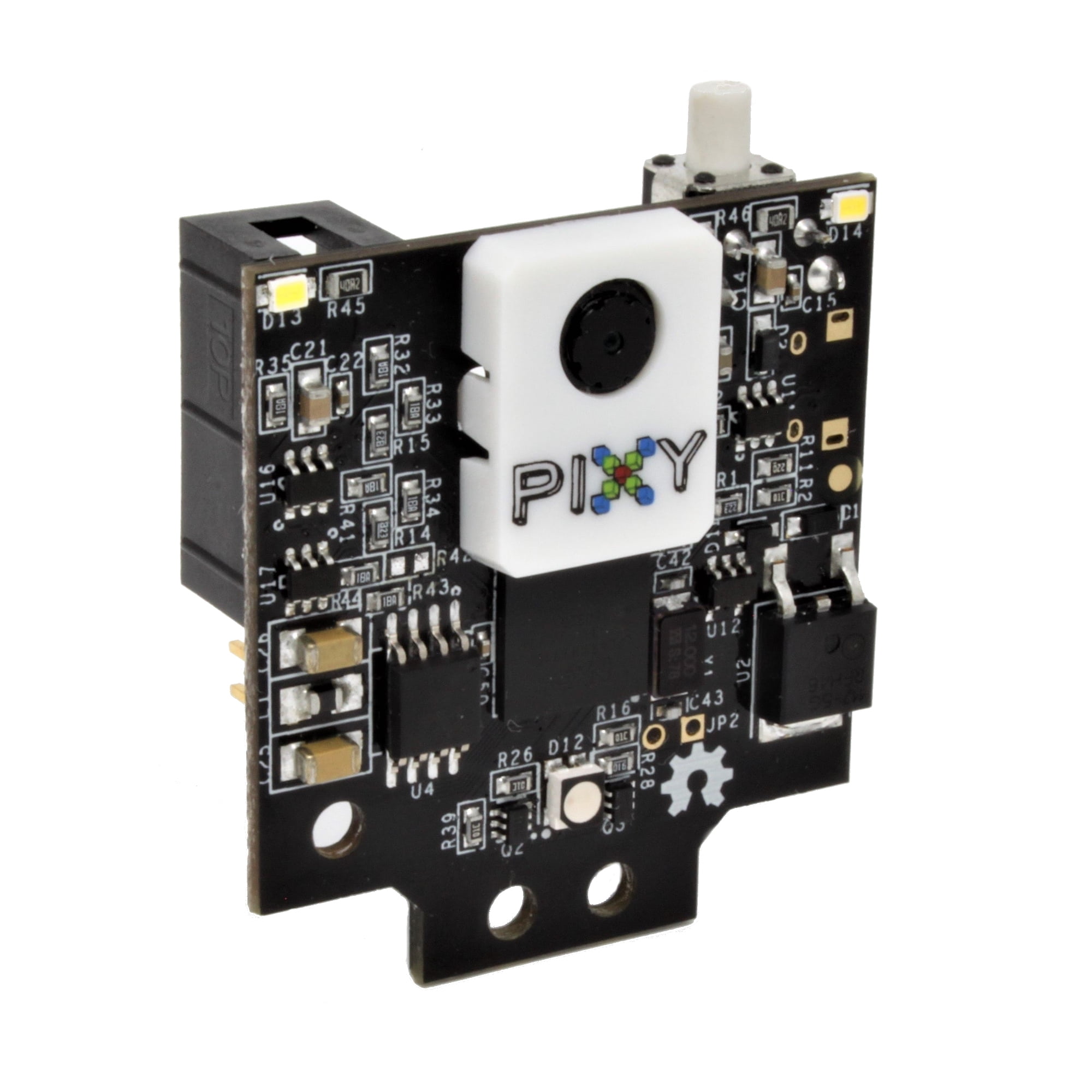

The speed is regulated using two heuristics: current deviation to the line center (which is the error) and how sharp is the line curve. Once we have processed the image and computed our deviation from the center of the line, we need to correct our error by regulating the car direction and speed.įor that purpose, we used a classic proportional-derivative (PD) controller to follow the line. The network was trained in less than 20 minutes on a 8-core laptop CPU. The hyperparameters details can be found in the appendix. We kept the model with the lowest error on the validation set and estimated our generalization error using the test set. To validate the hyper-parameters choice, we split the dataset into 3 subsets: a training set (60%), a validation set (20%) and a test set (20%). Hyperparameters include the network architecture, the learning rate, the minibatch size, … By contrast, the values of other parameters are derived via training. Hyperparameters are parameters whose values are set prior to the commencement of the learning process. Although we experimented other architectures, including CNNs, this one achieved good results and could run in real time at more than 60 FPS! Hyperparameters We used a feed forward neural network composed of two hidden layers with respectively 8 and 4 units. To increase the number of training samples, we flipped vertically the images, multiplying the size of the training set by 2 in a quick and cheap way. The preprocessing script can be found below: In our case, we normalized the input images in the range and scaled the output (predicted x-coordinate of the center) to. To avoid learning issues and speed up training, it is a good practice to normalize the data. That simplifies the problem and accelerates both training and prediction time.
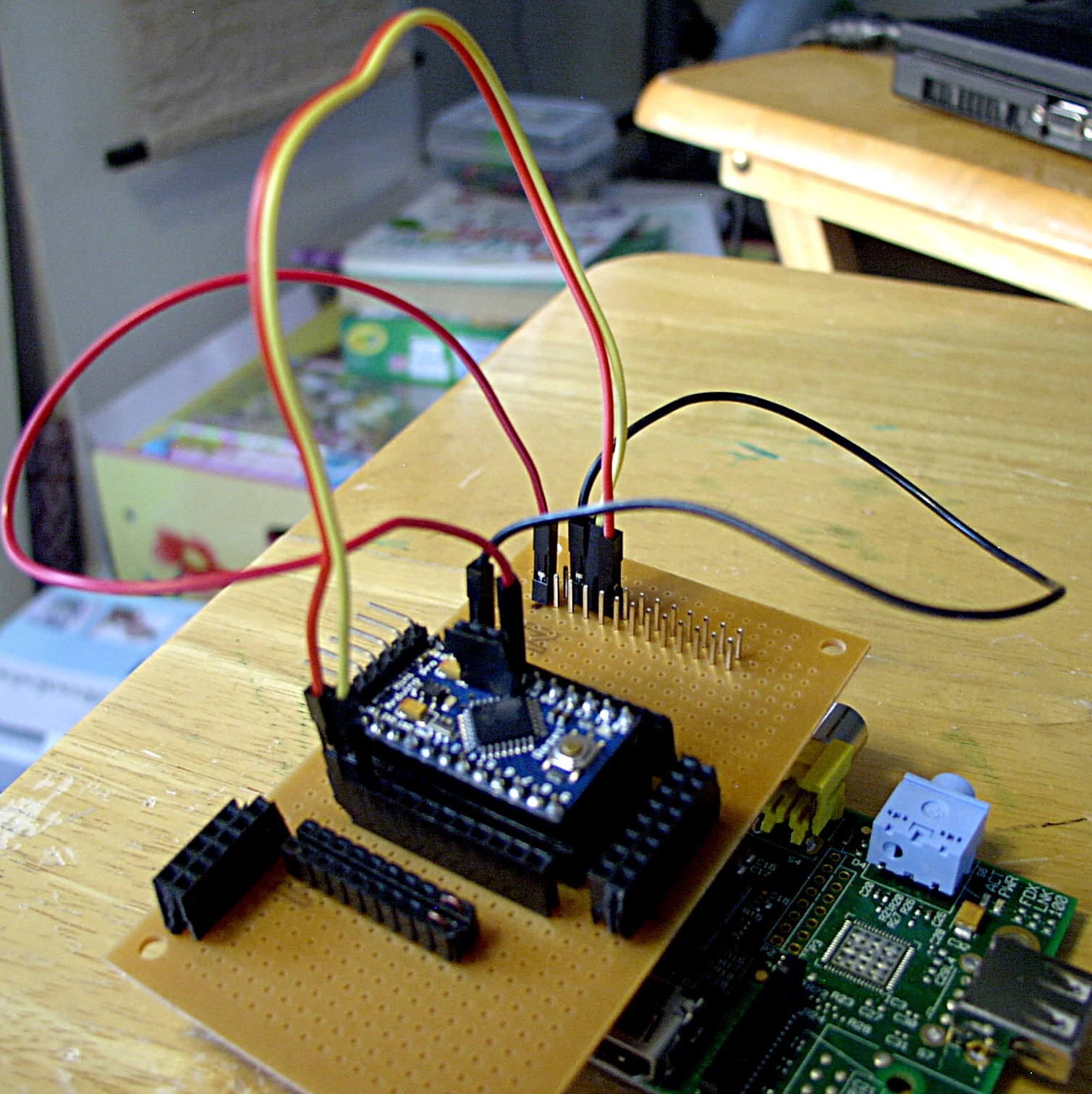
First, we resize the input images to reduce the input dimension (by a factor of 4), it allows to drastically cut down the number of learned parameters. Several steps are required before applying our learning algorithm on the data. For that purpose, we created our own labeling tool: each training image is shown one by one, we have to click on the center of the white line and then press any key to pass to the next image. Image LabelingĪfter recording a video in remote control mode, we manually labeled 3000 images (in ~25 minutes, i.e. To evaluate how good is our model, we chose the Mean Squared Error (MSE) loss as the objective: we take the squared error between the x-coordinate of the predicted and the true line center and average it over all the training samples. we assumed that the center is located at half of the height of the cropped image. We simplified the problem by predicting only the x-coordinate (along the width) of the line center, given a region of the image, i.e. In our case, we wanted to predict the coordinates of the line center given an input image from the camera. predict if an image contains a cat or a dog). In a supervised learning setting, that is to say, when we have labeled data, the goal is to predict the label given the input data (e.g. I chose to use a neural network because that was the method I’m the most familiar with and it was easy to implement using pure numpy and python code. So, we decided to apply machine learning to detect the line, that is to say we wanted to train a model than can predict where is the line given an image as input. We tried histogram equalization to overcome this issue but this was not sufficient and computationally costly. Which instruction are you following? (Is it our paper instruction manual, online documentation, or video tutorial? A link will Help.The main drawback of the previous method is that it is not robust to illumination changes.Which Platform are you using the product(s) on? (Is it a Raspberry Pi 3B+/4B, Arduino UNO R3, Jetson Nano B01, or another host?).What’s the Model number of the product(s) you’ve purchased? (If you don’t know the model number, show us the link to the product.).Which seller did you purchase the product(s) from? (Is it Amazon, UCTRONICS, or other Arducam distributors?).If you need help with the Arducam products you’ve purchased, please include the following questions in your post and answer them to help us better understand your needs. If you prefer a private conversation with Arducam, go to our Contact Center. The posting rules aim to help you better articulate your questions and be descriptive enough to get help.Īny topic that fails to comply with the posting rules will be unapproved starting from. Here are our forum rules to comply with if you want to post a new topic: Arducam posting rules
